Understanding Product Analytics - Unlocking Insights and Driving Growth
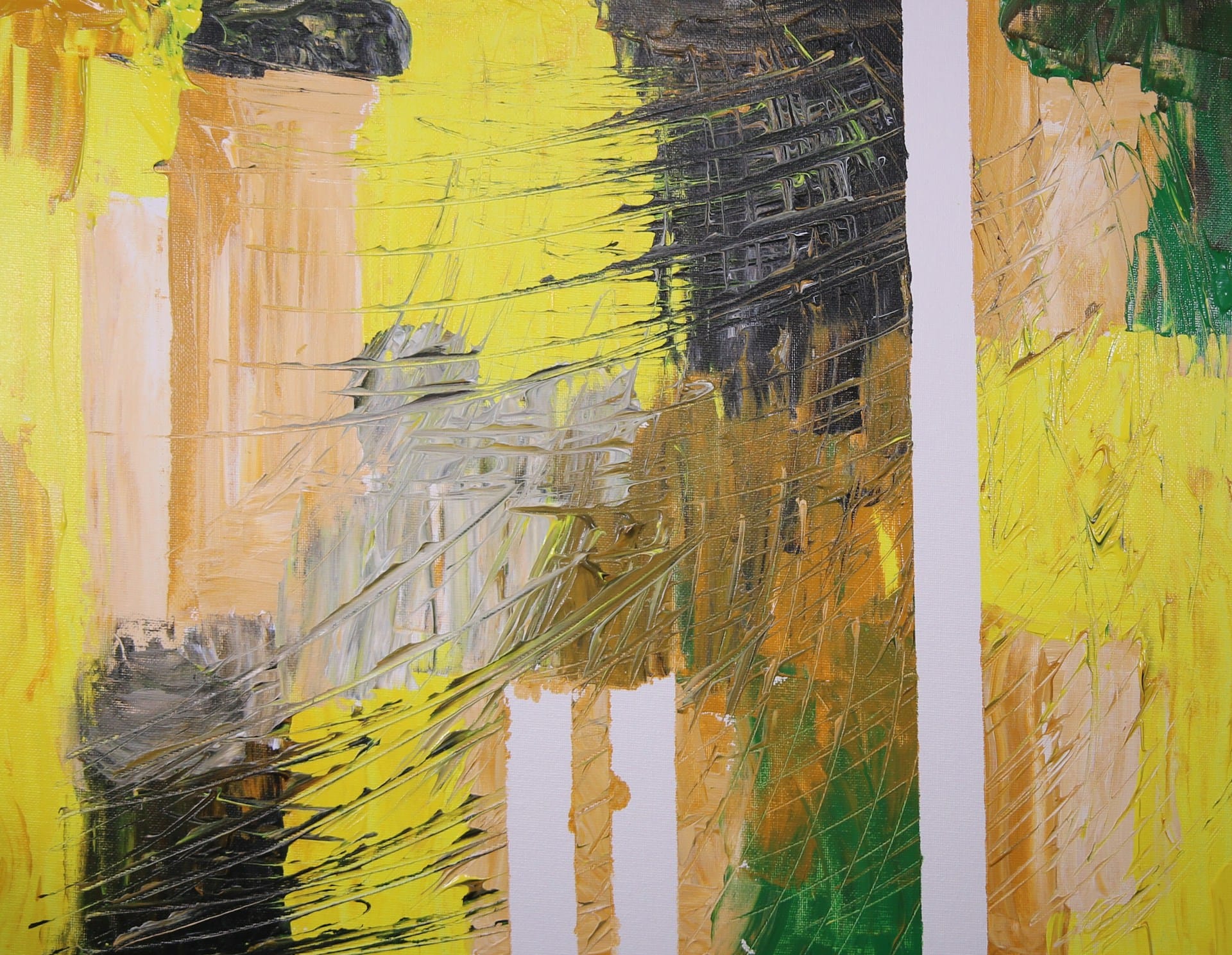
Product Analytics involves systematically examining data generated by users interacting with a product, aiming to understand user behavior, measure performance, and inform product decisions. It plays a crucial role in helping businesses enhance user experiences, optimize product features, and drive growth by leveraging insights drawn from user data. The product analytics process encompasses the collection, analysis, and interpretation of data, enabling companies to make data-driven decisions that align with their strategic objectives. Ultimately, effective product analytics leads to more informed product development, improved customer satisfaction, and increased business success.
Setting Objectives and Goals
Defining Business Objectives: Clearly outline the primary goals your business aims to achieve with its product, such as increasing user engagement, boosting sales, or improving customer retention.
Identifying Key Performance Indicators (KPIs): Determine the specific metrics that will be used to measure progress toward your business objectives, such as DAU (Daily Active Users), MAU (Monthly Active Users), conversion rates, or churn rates.
Aligning Analytics Goals with Business Strategy: Ensure that the goals set for product analytics are in harmony with the overall business strategy, providing a cohesive approach to achieving desired outcomes.
Data Collection
Types of Data: Collect qualitative data (user feedback, surveys) and quantitative data (clicks, session duration) to get a comprehensive view of user behavior and preferences.
Data Sources: Utilize various sources like user behavior tracking, customer feedback, and sales data to gather relevant information about product usage and performance.
Tools for Data Collection: Employ tools such as Google Analytics, Mixpanel, and Amplitude to systematically collect and organize data for analysis.
Data Management
While most Product Managers are not directly involved in data management, it is important to understand the key concepts in managing data.
Data Storage Solutions: Data storage solutions like data warehouses (e.g., Amazon Redshift, Google BigQuery) and cloud storage (e.g., AWS S3, Google Cloud Storage) are critical for housing vast amounts of collected data. These solutions offer scalability, allowing businesses to handle increasing data volumes as their user base grows, and ensure that data is accessible for analysis when needed.
Data Cleaning and Preprocessing: Data cleaning involves identifying and rectifying errors or inconsistencies in the dataset, such as missing values or duplicates, to improve data quality. Preprocessing prepares the data for analysis by transforming raw data into a suitable format, which may involve normalization, categorization, and handling outliers, ensuring the dataset is ready for accurate analysis.
Ensuring Data Quality and Integrity: Maintaining data quality involves continuous monitoring and validation to ensure data remains accurate, consistent, and reliable over time. Implementing data governance policies and using tools for data quality assessment (e.g., Talend, Informatica) helps preserve data integrity, which is essential for making trustworthy business decisions based on analytics.
Data Analysis Techniques
Descriptive Analytics: This technique involves summarizing historical data to understand what has happened in the past, using methods such as statistical analysis and data visualization to provide a clear picture of past trends and patterns.
Diagnostic Analytics: This approach focuses on investigating the reasons behind past outcomes by analyzing data correlations and patterns, helping to identify the root causes of specific events or behaviors.
Predictive Analytics: Utilizing machine learning and statistical models, predictive analytics forecasts future trends and outcomes based on historical data, aiding in proactive decision-making and strategy formulation.
Prescriptive Analytics: This advanced technique recommends specific actions to achieve desired outcomes, using optimization and simulation algorithms to suggest the best course of action based on the data analysis.
User Behaviour Analysis
Tracking User Interactions: Monitor and record user activities, such as clicks, page views, and session durations, to gain insights into how users interact with the product and identify areas for improvement.
Analyzing User Journeys and Funnels: Examine the paths users take through the product and the stages where they drop off, helping to optimize user flow and increase conversion rates.
Identifying User Segments and Personas: Categorize users into distinct segments based on their behavior and characteristics, creating detailed personas to tailor marketing strategies and product features to specific user needs.
Product Performance Metrics
User Engagement Metrics: Metrics like Daily Active Users (DAU), Monthly Active Users (MAU), and session duration provide insights into how frequently and how long users interact with the product, helping to scale the product’s ability to retain users and overall user interest.
Conversion Metrics: Metrics such as conversion rates (percentage of users completing a desired action) and drop-off rates (points where users abandon a process) are crucial for understanding the effectiveness of the product in driving user actions and identifying obstacles in the user journey.
Revenue Metrics: Key metrics like Average Revenue Per User (ARPU), Customer Lifetime Value (LTV), and Customer Acquisition Cost (CAC) help measure the financial performance of the product, ensuring that the product contributes positively to the company's bottom line and identifying areas for revenue optimization.
A/B Testing and Experimentation
Designing Experiments: Carefully plan experiments by defining hypotheses, selecting target user groups, and determining the variables to test, ensuring that the tests are structured to yield meaningful insights.
Running A/B Tests: Conduct A/B tests by comparing two or more versions of a product feature with different user groups to identify which version performs better in terms of defined metrics, such as engagement or conversion rates.
Analyzing Results and Implementing Changes: Analyze the outcomes of A/B tests to draw conclusions about user preferences and the effectiveness of changes, then implement successful variations to optimize the product based on data-driven evidence.
Visualization and Reporting
Tools for Data Visualization: Utilize platforms such as Tableau, Power BI, and Looker to create visually engaging dashboards and reports that facilitate the interpretation of complex data sets, enabling stakeholders to grasp insights quickly and make informed decisions.
Creating Dashboards and Reports: Design intuitive dashboards and reports that present key metrics and trends in a clear and concise manner, allowing stakeholders to monitor performance and track progress toward goals effectively.
Communicating Insights to Stakeholders: Present findings and recommendations in a compelling and accessible way, tailoring the communication to the audience's level of expertise and ensuring that insights are actionable and drive decision-making across the organization.
Implementing Insights
Turning Insights into Action: Translate data-driven insights into concrete actions by prioritizing product improvements, allocating resources effectively, and implementing changes that align with strategic objectives.
Tracking the Impact of Changes: Continuously monitor key metrics and performance indicators to assess the impact of implemented changes, iterate on strategies based on feedback, and optimize the product iteratively for ongoing improvement.
Pitfalls to Avoid While Analyzing Data
Ignoring Business Objectives: Focusing solely on data analysis without aligning it with the company's strategic goals can lead to irrelevant insights.
Overlooking Data Quality: Relying on incomplete, inaccurate, or biased data can result in misleading conclusions and poor decision-making.
Analysis Paralysis: Getting bogged down in endless data exploration without taking action can delay decision-making and hinder progress.
Lack of Stakeholder Communication: Failing to communicate findings effectively to stakeholders can result in misalignment and resistance to change.
Disregarding User Context: Analyzing data without considering user context and qualitative feedback can lead to misinterpretation and misguided strategies.
If Nothing Else, Remember This😉
- Align product analytics with business objectives to ensure actionable insights.
- Prioritize accurate and comprehensive data collection and analysis for reliable insights.
- Incorporate both quantitative and qualitative data to understand user behavior effectively.
- Continuously analyze and iterate based on data to optimize product performance.
- Ensure clear and frequent communication of insights to stakeholders to drive informed decision-making.
Thanks for reading, hope that you find this content valuable!! Please do share this with your friends & colleagues and subscribe to our weekly posts.